ChatGPT as a Grammar Checker and Editor
Introduction
A popular use case for LLMs is as a writing assistant, for example for editing text as a grammar and spelling checker. Students may need help with writing essays, while businesses use it for drafting and editing emails, marketing copy, reports, etc.
An early adopter of language models for writing assistance was Google within the Gmail product. With broader availability of LLM platforms for lower costs, major companies have integrated LLMs into their products for writing assistance, such as Apple and Microsoft.
However, many of these systems present a popup and do not display suggestions inline.
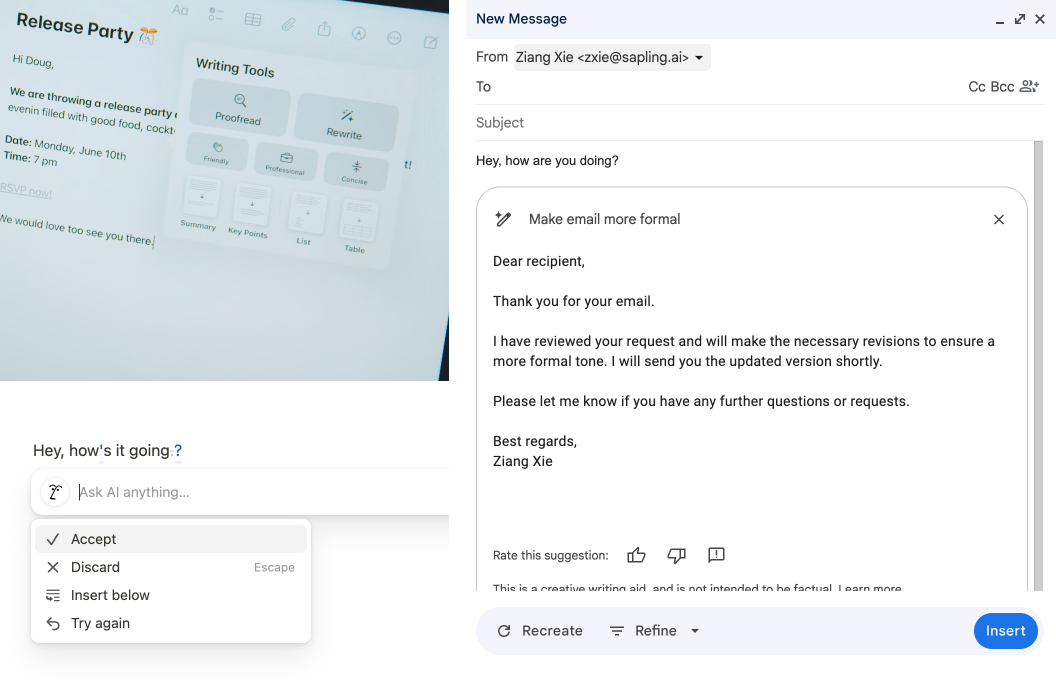
There has been a gap between the ability of LLMs to generate text and the tight integration of LLMs within text editors. We consider why this is the case, taking the current most popular frontier LLM, ChatGPT-4o mini, as an example, and explain why you should consider using a task-specific LLM for grammar checking (such as the one offered by Sapling's API).
Limitations of LLMs
While LLMs are now powerful assistants for Q&A, they often do not generate text in a specific format. For example, OpenAI must enforce that models follow a Structured Output schema as a separate model capability.
For the task of text editing, the simplest output is just the corrected version of the text (the output could also be a list of edits with the edit positions, but this is more expensive in terms of token count, and many LLMs are not great at providing exact numbers without invoking separate modules).
During pretraining, LLMs are simply trained to predict the next word in a sequence. In order to perform well at the chat / Q&A task, LLMs are then instruction fine-tuned so that they respond with a helpful answer instead of just a continuation. To consistently attain an exact output format, either a long prompt or finetuning is needed.
Take the following conversations as examples:
[All conversations were with ChatGPT-4o mini on 2024-11-14
with no previous context or system prompt.]
Example 1
Human: Correct the grammar: Hi how are you doing
Assistant: The corrected sentence is: “Hi, how are you doing?”
This results in the extraneous phrase "The corrected sentence is:" at the beginning of the response.
Example 2
Human: Correct the grammar: Let me see what we can
Assistant: Certainly! A grammatically complete version of the phrase would be:
“Let me see what we can do.”
This completes the thought and makes the sentence clear and grammatically correct.
There is unnecessary text preceding and following the output sentence. The output sentence includes an autocompletion which may or may not be what was desired.
Example 3
Human: Correct the grammar: Hope all's well
Assistant: The grammatically correct version would be: “I hope all is well.”
The casual statement "Hope all's well" may very well be what the user intended, especially say in a less formal email response.
Workarounds
There are two methods to mitigate the above issues:
- Provide more detailed instructions in the prompt.
- Finetune the system on the editing task.
The first method is easier to get started with, but can be costly as the prompt may often be longer than the input text.
Human: You are a grammar assistant for real-time editing.
Do not complete partial sentences.
Also, do not provide unnecessary edits for casual statements.
Only output the corrected text.
Now correct the following text: How's it going?
Assistant: How's it going?
The second method requires some data collection, and also doubles the cost of each API call
(as of this writing, for GPT-4o mini, this is $0.300 / 1M
input tokens and $0.600 / 1M
output tokens).
Deployment Considerations
Outside of the output format, here are some other considerations prior to deploying LLMs for the editing task.
Benchmarking
We highly recommend benchmarking the performance of the system on a real-world dataset prior to deployment. You can find our guidelines for this here. The intuition is that intentional errors made by developers and administrators doing testing are very different from accidental errors made by users. During testing, false negatives are the emphasis, while during production, false positives get annoying very quickly.
Latency
While LLMs have become very fast, there is still often a significant delay (time to first token) and processing time (tokens per second). Fortunately, for editing latency is not as critical of a requirement as for autocompletion. Even so, latencies of well under a second are desirable for real-time editing.
Refusals
Surprisingly, sometimes even frontier LLMs will have guardrails in place that prevent them from making recommendations. This can occur, for example, when the text contains offensive language or content.
Postprocessing
Once you have the corrected text, you'll still need to compute the diff between the old and new text to obtain the edit positions. This is simple for small edits, but for a paragraph where there may be cross-sentence edits, this may not be do-able with a plain edit distance-based method. Sapling's backend handles this for you.
Customization Options
Language is not one-size-fits-all. We previously mentioned the example of casual vs. formal English. Another example is that you may need to handle different English varieties (US/UK/AU/CA/etc.). Different businesses may also have their own style guides. Despite learning-based systems greatly outperforming rule-based systems in their ability to generalize, most editing use cases still involve some deterministic logic.
Displaying Edits
Lastly, you'll need to display the edits to the user, which may require significant time from a frontend developer. This is especially true if you wish to display the edits overlaid on top of the editable text.
Sapling's SDK allows you to attach grammar checking functionality to any text input field (textarea
or contenteditable
) with a few lines of code.
Conclusion
We hope this has been a helpful guide for how you may use ChatGPT as a grammar checker. In case you face some of the challenges presented above, Sapling's API offers a task-specific LLM for grammar checking that may be a good fit. Depending on usage volume, our pricing is competitive with major LLM providers.
As of this writing, on our benchmark of 5000 sentences, Sapling's API also significantly outperforms GPT-4o mini on the industry-standard F0.5-score metric (contact us for more details).
Contact us at sales@sapling.ai or through our contact form here for more information.